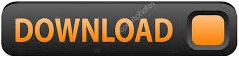
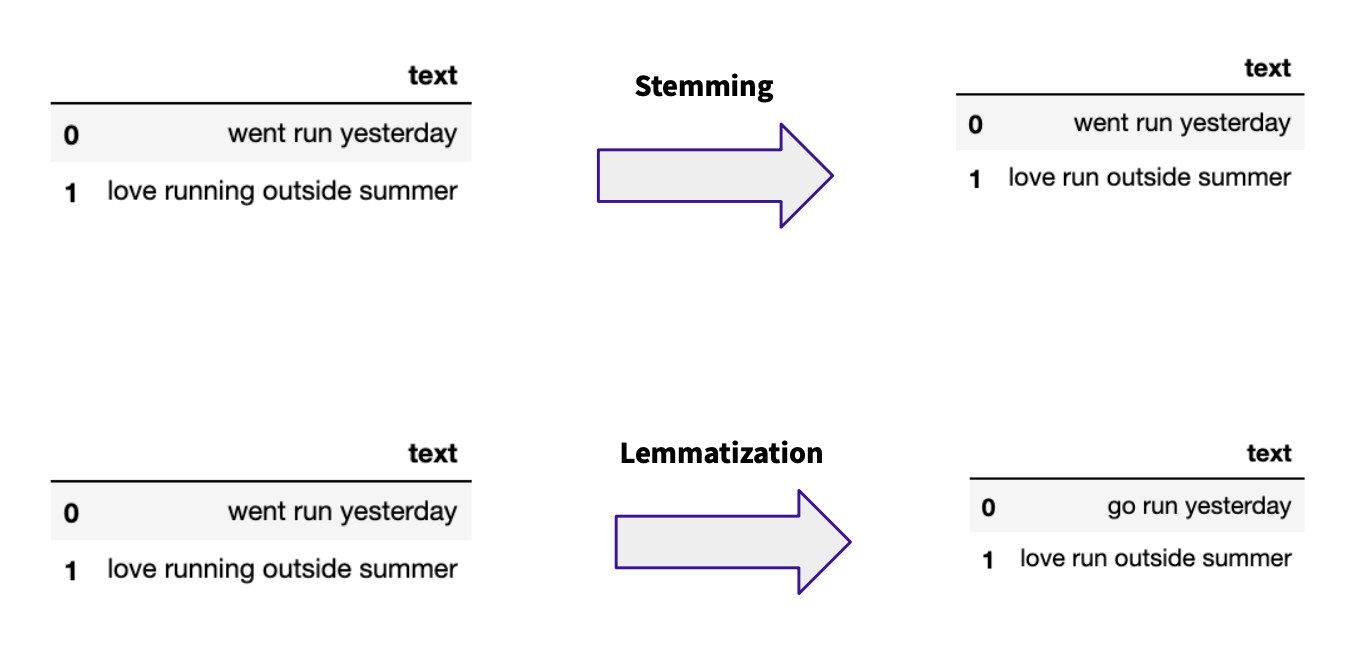
Stemming and Lemmatization: Stemming is the process of removing characters from the beginning or end of a word to reduce it to their stem.Depending on the desired outcome, correcting spelling errors or not is a critical step. Official corporate or education documents most likely contain fewer errors, where social media posts or more informal communications like email can have more. Depending on the medium of communication, there might be more or fewer errors.
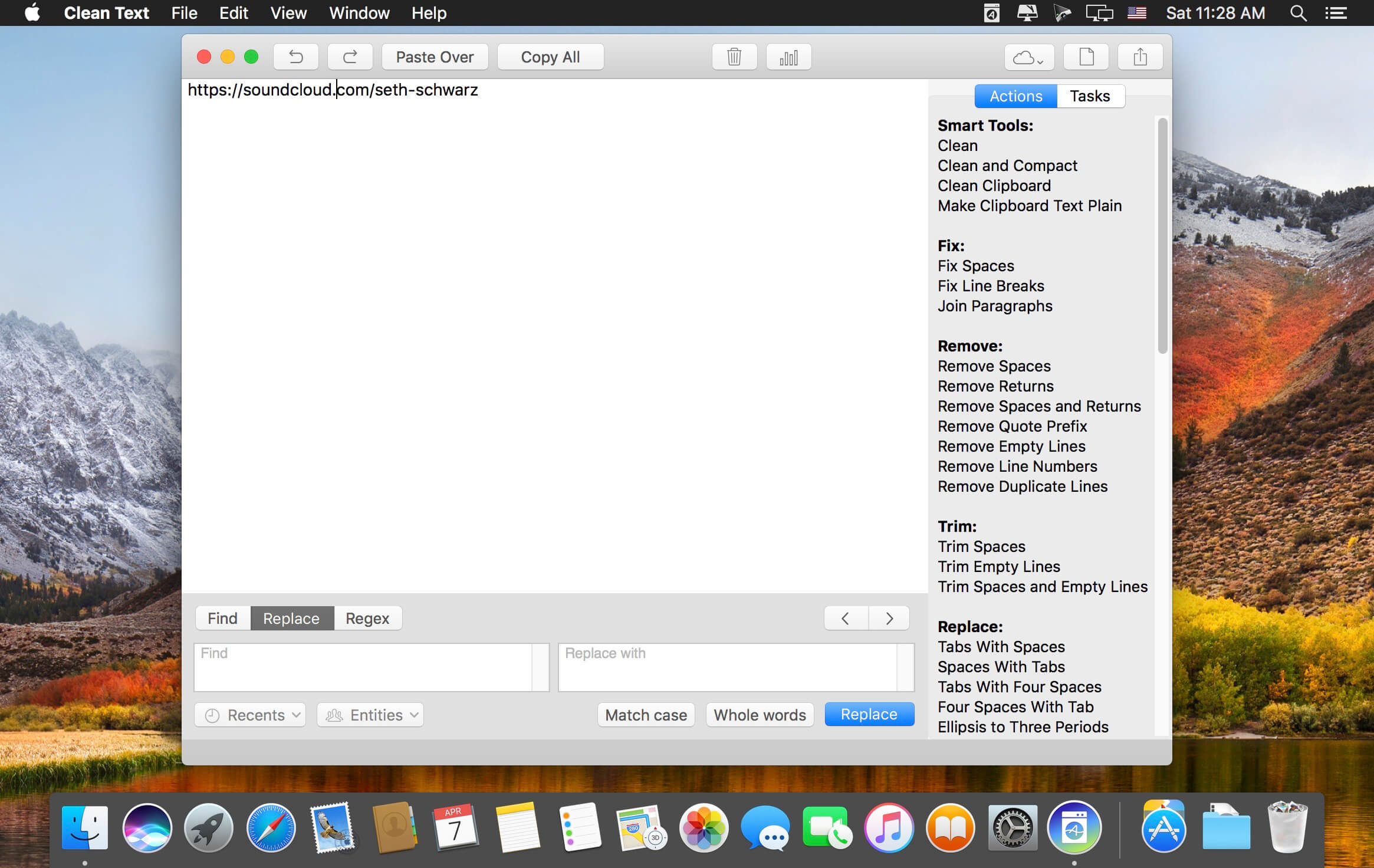
#Nlp clean text software
Most software packages handle edge cases (U.S. Special care has to be taken when breaking down terms so that logical units are created. We understand these units as words or sentences, but a machine cannot until they’re separated. Tokenization: Tokenization breaks the text into smaller units vs.The following are general steps in text preprocessing: This post will show how I typically accomplish this. In order to maximize your results, it's important to distill you text to the most important root words in the corpus. One of the most common tasks in Natural Language Processing (NLP) is to clean text data.
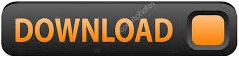